IPAdapter V1 FaceID Plus | Consistent Characters
Unlock the full potential of your character designs with the IPAdapter Face Plus V2 model. This workflow allows creators to maintain consistent character features across various styles. Feel free to use different checkpoints or LoRA models to explore a variety of stylesComfyUI Consistent Characters Workflow
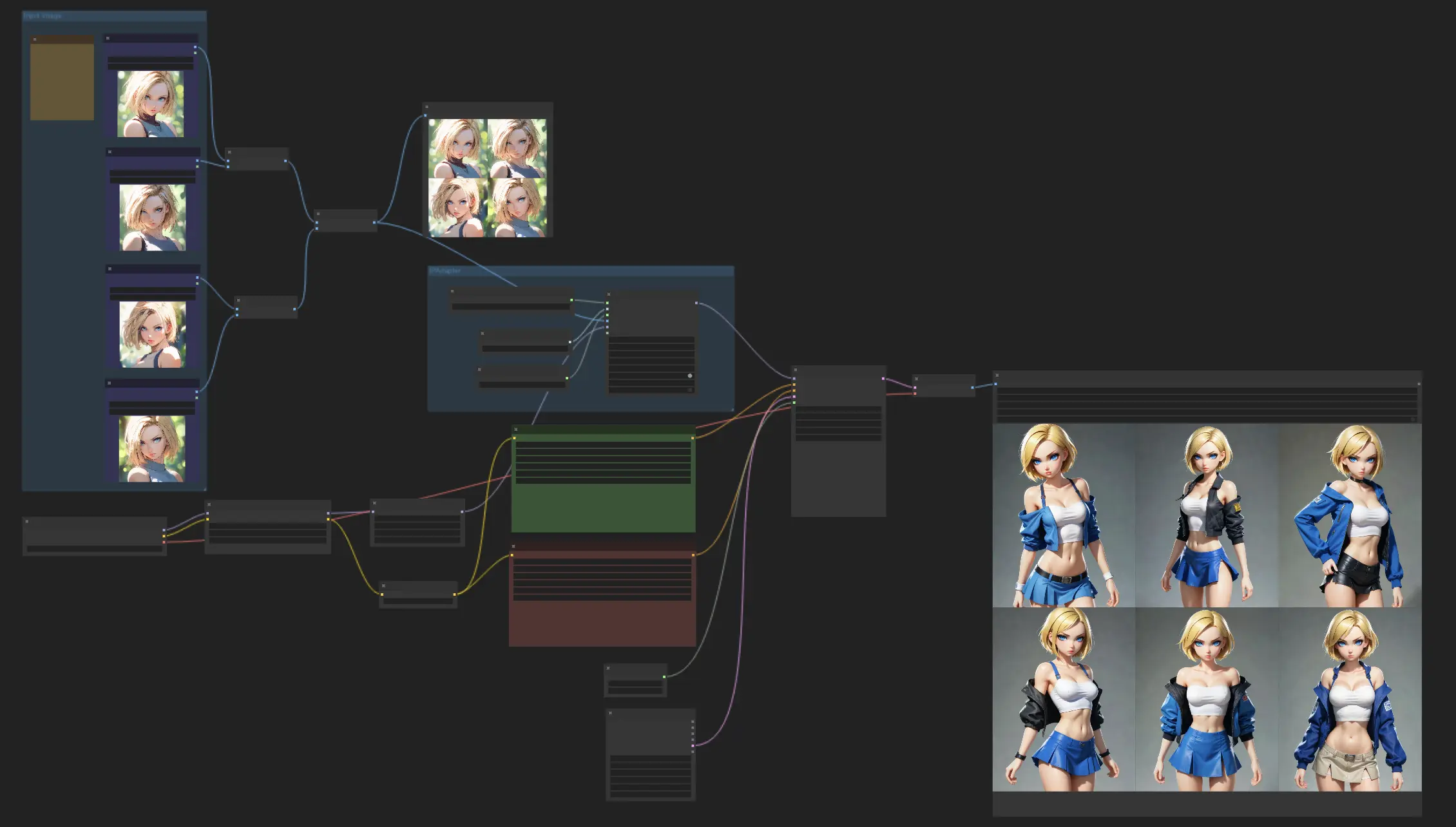
- Fully operational workflows
- No missing nodes or models
- No manual setups required
- Features stunning visuals
ComfyUI Consistent Characters Examples
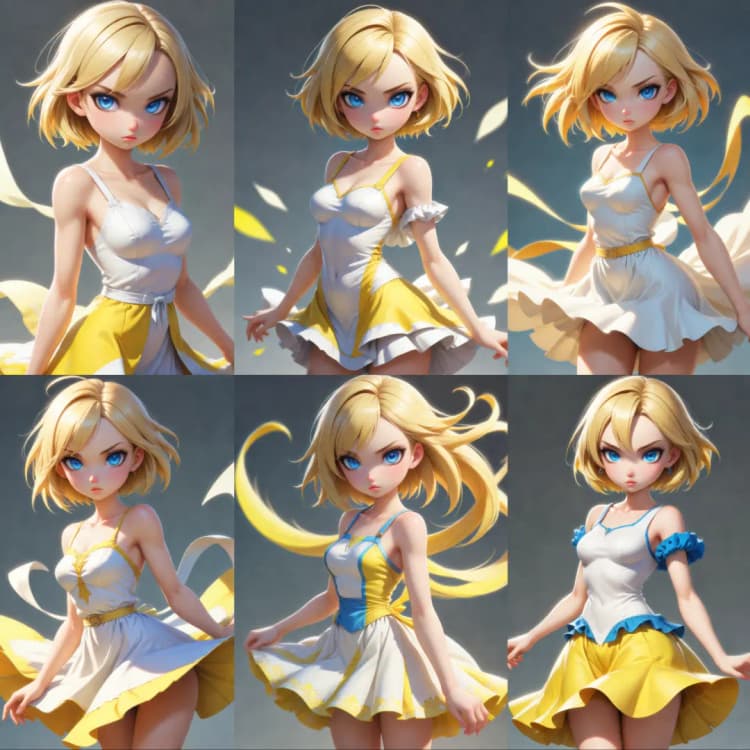
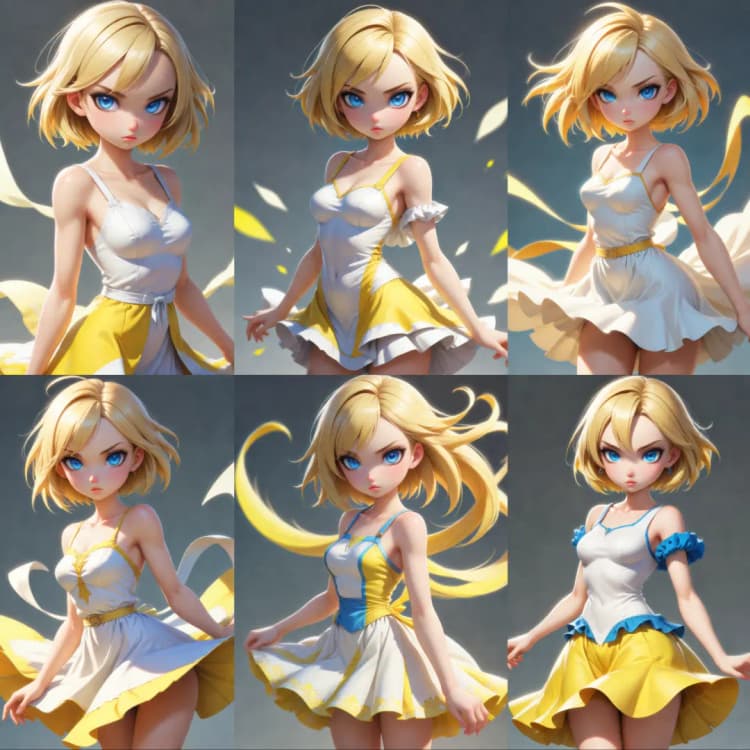
ComfyUI Consistent Characters Description
1. Consistent Character Workflow
This workflow is all about crafting characters with a consistent look, leveraging the IPAdapter Face Plus V2 model. Simply start by uploading some reference images, and then let the Face Plus V2 model work its magic, creating a series of images that maintain the same facial features. Feel free to mix things up with different checkpoints or LoRA models to explore a variety of styles, all while keeping your character's appearance consistent.
2. Overview of IPAdapter FaceID/FaceID Plus
v1.5 FaceID
This model is the base version for face identification, allowing for variations augmented by text prompts, control nets, and masks. It's noted for its average strength in conditioning, making it suitable for general face conditioning tasks. The base FaceID model does not utilize a CLIP vision encoder, which implies a simpler setup without the need for complex encoder configurations.
v1.5 FaceID Plus
The FaceID Plus model is a more potent variant, designed for stronger image-to-image conditioning effects. It requires the use of the ViT-H image encoder, indicating its need for higher processing capabilities for detailed face modeling.
v1.5 FaceID Plus v2
An iteration over the FaceID Plus, this model introduces enhancements for even more detailed face conditioning. Similar to FaceID Plus, it utilizes the ViT-H image encoder. This model aims at providing an increased quality in face modeling, catering to more nuanced requirements.
v1.5 FaceID Portrait
Designed specifically for portraits, this model does not use a CLIP vision encoder. It focuses on generating high-quality facial images within portrait settings, potentially offering a specialized approach for portrait image generation.
SDXL FaceID
The SDXL variant of FaceID is tailored for use with the SDXL architecture, not employing a CLIP vision encoder. It represents a base model within the SDXL suite, designed for scalable deep learning architectures, focusing on face identification tasks.
SDXL FaceID Plus v2
This is a stronger version of the FaceID model for the SDXL architecture, utilizing the ViT-H image encoder. It's designed to offer enhanced face conditioning effects within the SDXL framework, aimed at high-quality image generation tasks.
3. How to use IPAdapter FaceID/FaceID Plus
3.1. Choose FaceID/FaceID Plus model
Select your preferred FaceID or FaceID Plus model to start crafting your images. Within the settings, you'll find options to adjust both the weights and the noise. These adjustments are key to fine-tuning the appearance of your generated images, allowing you to achieve the precise look you're aiming for.
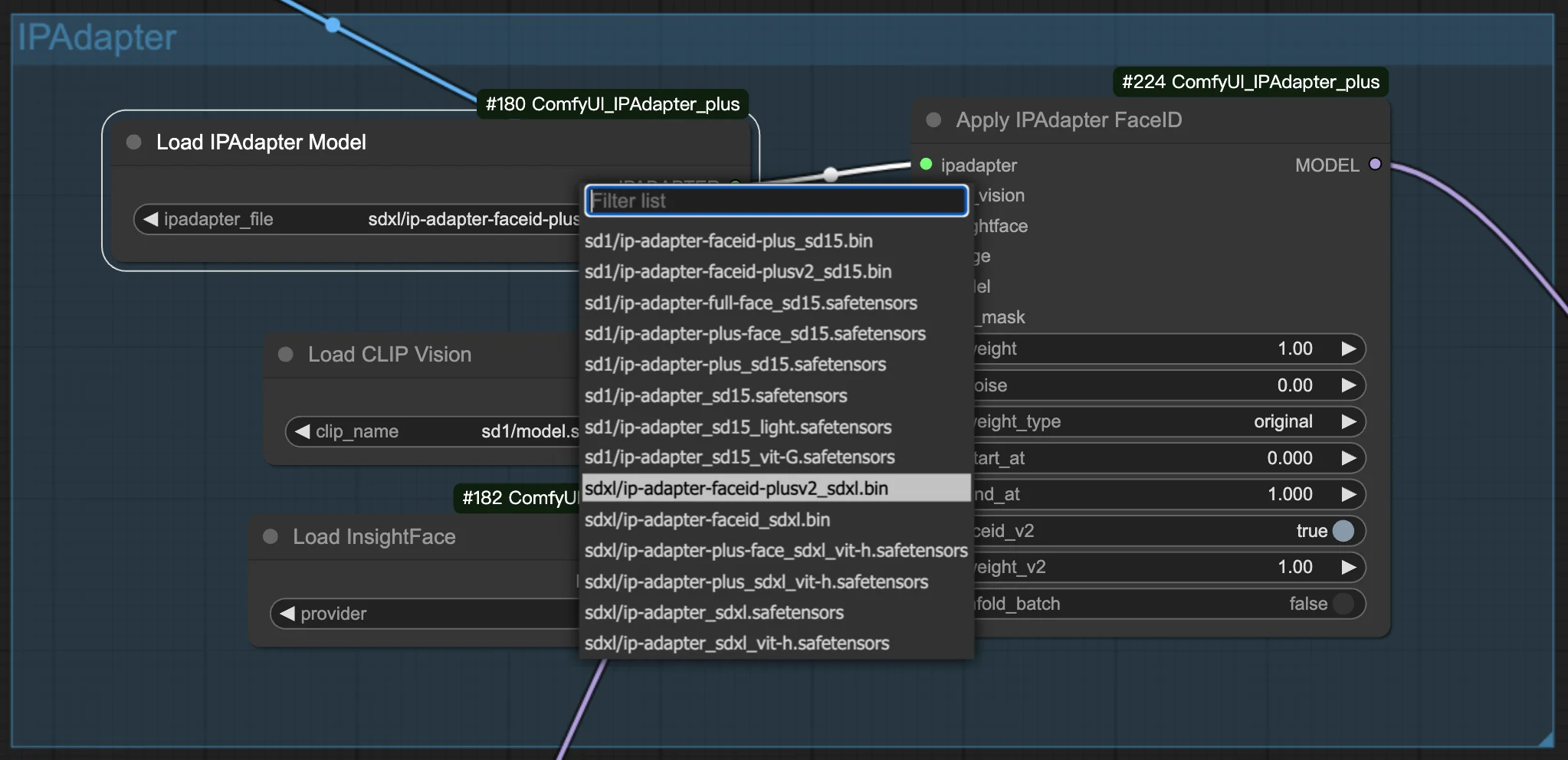
3.2. Preparing the reference image
When using IPAdapter FaceID nodes, the CLIP vision model processes your reference image by resizing and centering it to a dimension of 224x224 pixels. This automatic adjustment focuses on the image's center, making it crucial for the main subject of your image, like a character's face, to be positioned centrally. If the subject is off-center, especially in portrait or landscape images, the results might not meet your expectations. For best outcomes, it's highly recommended to use square images with the subject centered.
Want More ComfyUI Workflows?
RunComfy is the premier ComfyUI platform, offering ComfyUI online environment and services, along with ComfyUI workflows featuring stunning visuals. RunComfy also provides AI Playground, enabling artists to harness the latest AI tools to create incredible art.